Publications
年轻人你的职责是平整土地,而非焦虑时光
你做三四月的事情,在八九月自有答案
——系外行星的研究僧
Journal Papers (published/accepted) 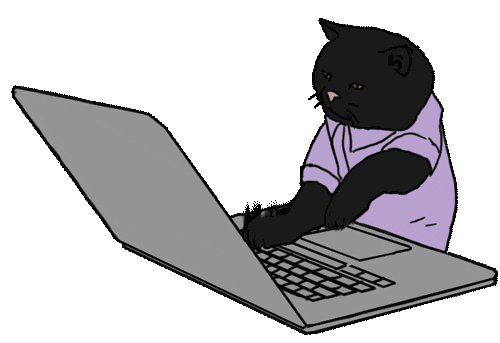
-
[IEEE JBHI] Wanyong Qiu, Yifan Feng, Yuying Li, Yi Chang, Kun Qian*, Bin Hu*, Yoshiharu Yamamoto, and Björn W. Schuller, "Fed-MStacking: Heterogeneous Federated Learning with Stacking Misaligned Labels for Abnormal Heart Sound Detection", IEEE Journal of Biomedical and Health Informatics (IF-2023: 6.7), vol. 28, no. 9, July 2024 (SCI 1区 Top).
PDF
Technical Report
show/hide abstract
Ubiquitous sensing has been widely applied in smart healthcare, providing an opportunity for intelligent heart sound auscultation. However, smart devices contain sensitive information, raising user privacy concerns. To this end, federated learning (FL) has been adopted as an effective solution, enabling decentralised learning without data sharing, thus preserving data privacy in the Internet of Health Things (IoHT). Nevertheless, traditional FL requires the same architectural models to be trained across local clients and global servers, leading to a lack of model heterogeneity and client personalisation. For medical institutions with private data clients, this study proposes Fed-MStacking, a heterogeneous FL framework that incorporates a stacking ensemble learning strategy to support clients in building their own models. The secondary objective of this study is to address scenarios involving local clients with data characterised by inconsistent labelling. Specifically, the local client contains only one case type, and the data cannot be shared within or outside the institution. To train a global multi-class classifier, we aggregate missing class information from all clients at each institution and build meta-data, which then participates in FL training via a meta-learner. We apply the proposed framework to a multi-institutional heart sound database. The experiments utilise random forests (RFs), feedforward neural networks (FNNs), and convolutional neural networks (CNNs) as base classifiers. The results show that the heterogeneous stacking of local models performs better compared to homogeneous stacking.
-
[IEEE TBME] Wanyong Qiu, Chen Quan, Lixian Zhu, Yongzi Yu, Zhihua Wang, Yu Ma, Mengkai Sun, Yi Chang, Kun Qian*, Bin Hu*, Yoshiharu Yamamoto, and Björn W. Schuller, "Heart Sound Abnormality Detection From Multi-Institutional Collaboration: Introducing a Federated Learning Framework", IEEE Transactions on Bio-medical Engineering (IF-2023: 4.7), vol. 71, no. 10, May 2024 (SCI 2区).
PDF
Technical Report
show/hide abstract
Objective: Early diagnosis of cardiovascular diseases is a crucial task in medical practice. With the application of computer audition in the healthcare field, artificial intelligence (AI) has been applied to clinical non-invasive intelligent auscultation of heart sounds to provide rapid and effective pre-screening. However, AI models generally require large amounts of data which may cause privacy issues. Unfortunately, it is difficult to collect large amounts of healthcare data from a single centre. Methods: In this study, we propose federated learning (FL) optimisation strategies for the practical application in multi-centre institutional heart sound databases. The horizontal FL is mainly employed to tackle the privacy problem by aligning the feature spaces of FL participating institutions without information leakage. In addition, techniques based on deep learning have poor interpretability due to their “black-box” property, which limits the feasibility of AI in real medical data. To this end, vertical FL is utilised to address the issues of model interpretability and data scarcity. Conclusion: Experimental results demonstrate that, the proposed FL framework can achieve good performance for heart sound abnormality detection by taking the personal privacy protection into account. Moreover, using the federated feature space is beneficial to balance the interpretability of the vertical FL and the privacy of the data. Significance: This work realises the potential of FL from research to clinical practice, and is expected to have extensive application in the federated smart medical system.
-
[AAAS CBS] Wanyong Qiu, Chen Quan, Yongzi Yu, Eda Kara, Kun Qian*, Bin Hu*, Björn W. Schuller, and Yoshiharu Yamamoto, "Federated Abnormal Heart Sound Detection with Weak to No Labels", Cyborg and Bionic Systems (IF-2023: 10.5), vol. 5, Sep 2024 (JCR 1区).
PDF
Technical Report
show/hide abstract
Cardiovascular diseases are a prominent cause of mortality, emphasizing the need for early prevention and diagnosis. Utilizing artificial intelligence (AI) models, heart sound analysis emerges as a noninvasive and universally applicable approach for assessing cardiovascular health conditions. However, real-world medical data are dispersed across medical institutions, forming “data islands” due to data sharing limitations for security reasons. To this end, federated learning (FL) has been extensively employed in the medical field, which can effectively model across multiple institutions. Additionally, conventional supervised classification methods require fully labeled data classes, e.g., binary classification requires labeling of positive and negative samples. Nevertheless, the process of labeling healthcare data is time-consuming and labor-intensive, leading to the possibility of mislabeling negative samples. In this study, we validate an FL framework with a naive positive-unlabeled (PU) learning strategy. Semisupervised FL model can directly learn from a limited set of positive samples and an extensive pool of unlabeled samples. Our emphasis is on vertical-FL to enhance collaboration across institutions with different medical record feature spaces. Additionally, our contribution extends to feature importance analysis, where we explore 6 methods and provide practical recommendations for detecting abnormal heart sounds. The study demonstrated an impressive accuracy of 84%, comparable to outcomes in supervised learning, thereby advancing the application of FL in abnormal heart sound detection.
-
[IEEE TIM] Lixian Zhu, Wanyong Qiu, Yu Ma, Fuze Tian, Mengkai Sun, Zhihua Wang, Kun Qian*, Bin Hu*, Yoshiharu Yamamoto, and Björn W. Schuller, "LEPCNet: A lightweight End-to-End PCG Classification Neural Network Model for Wearable Devices", IEEE Transactions on Instrumentation & Measurement (IF-2023: 5.6), vol. 73, no. 2511111, Sep 2023 (SCI 2区 Top).
PDF
Technical Report
show/hide abstract
Wearable intelligent phonocardiogram (PCG) sensors provide a non-invasive method for long-term monitoring of cardiac status, which is crucial for the early detection of cardiovascular diseases. As one of the key technologies for intelligent PCG sensors, PCG classification techniques based on computer audition have been widely leveraged in recent years, such as Convolutional Neural Networks, Generative Adversarial Nets, and Long Short-Term Memory. However, the limitation of these methods is that the models have a sizeable computational complexity, which is not suitable for wearable devices. To this end, we propose an end-to-end neural network for PCG classification with low computational complexity (52.67K parameters and 1.59M floating point operations per second (FLOPs). We utilise two public datasets to test the model, and experimental results demonstrate that the proposed model achieves an accuracy of 93.1% in the 2016 PhysioNet/CinC Challenge dataset with considerable complexity reduction compared to the state-of-theart works. Moreover, we design an energy-efficient wearable PCG sensor and deploy the proposed algorithms on it. The experimental results show that our proposed model consumes only 245.1mW for PCG classification with an accuracy of 89.8% on test datasets. This means that the proposed model obtains excellent performance compared to previous work while consuming lower power, which is significant in practical application scenarios.
-
[IEEE JBHI] Xihang Qiu, Wanyong Qiu, Ye Zhang, Kun Qian*, Chun Li, Bin Hu*, Björn W. Schuller and Yoshiharu Yamamoto, "FedKDC: Consensus-Driven Knowledge Distillation for Personalized Federated Learning in EEG-Based Emotion Recognition", IEEE Transactions on Instrumentation & Measurement (IF-2023: 6.7), in press, April 2025 (SCI 1区 Top).
PDF
Technical Report
show/hide abstract
Federated learning (FL) has gained prominence in electroencephalogram (EEG)-based emotion recognition because of its ability to enable secure collaborative training without centralized data. However, traditional FL faces challenges due to model and data heterogeneity in smart healthcare settings. For example, medical institutions have varying computational resources, which creates a need for personalized local models. Moreover, EEG data from medical institutions typically face data heterogeneity issues stemming from limitations in participant availability, ethical constraints, and cultural differences among subjects, which can slow model convergence and degrade model performance. To address these challenges, we propose FedKDC, a novel FL framework that incorporates clustered knowledge distillation (CKD). This method introduces a consensus-based distributed learning mechanism to facilitate the clustering process. It then enhances the convergence speed through intraclass distillation and reduces the negative impact of heterogeneity through interclass distillation. Additionally, we introduce a DriftGuard mechanism to mitigate client drift, along with an entropy reducer to decrease the entropy of aggregated knowledge. The framework is validated on the SEED, SEED-IV, SEED-FRA, and SEED-GER datasets, demonstrating its effectiveness in scenarios where both the data and the models are heterogeneous. Experimental results show that FedKDC outperforms other FL frameworks in emotion recognition, achieving a maximum average accuracy of 85.2%, and in convergence efficiency, with faster and more stable convergence. Our code is made publicly available at: https://github.com/wdqdp/FedKDC.
-
[IEEE TCSS] Guihua Tian*, Kun Qian*, Xinyi Li, Mengkai Sun, Hao Jiang, Wanyong Qiu, Xiaoming Xie, Zhonghao Zhao, Liangqing Huang, Siyan Luo, Tianxing Guo, Ran Cai, Zhihua Wang, and Björn W. Schuller, "Can a Holistic View Facilitate the Development of Intelligent Traditional Chinese Medicine? A Survey", IEEE Transactions on Computational Social Systems (IF-2023: 4.5), vol. 10, no. 2, Sep 2023 (SCI 2区).
PDF
Technical Report
show/hide abstract
Intelligent traditional Chinese medicine (ITCM) is an emerging interdisciplinary subject. It aims to efficiently and precisely promote the prevention and treatment of diseases and health management in Chinese medicine clinical practice via the combination of traditional Chinese medicine (TCM) fundamentals and artificial intelligence technologies. Presently, it is experiencing dramatic growth in recent years. On the one hand, a holistic view, as a crucial philosophy in the theory of TCM, will be guiding the development of ITCM. On the other hand, a comprehensive discussion of the benefits of such a holistic view of ITCM is lacking. To this end, we conduct this survey by introducing the named holistic view first. Then, adaptive learning and field theory will be presented and discussed with respect to their application in ITCM. Ethical issues of ITCM will then be taken into account by human-centered TCM and potentials based on affective computing of ITCM. In addition, we give our opinions and insights on the challenges and open issues regarding the future of ITCM. We hope that this survey article can be a good guide for experts in the relevant fields.
-
[Journal of Cyber Security] 贾俊杰, 邱万勇*, 马慧芳, "差分隐私保护约束下集成分类算法的研究", 信息安全学报, vol. 6, no. 4, Sep 2021 (CCF A).
PDF
Technical Report
show/hide abstract
机器学习中的隐私保护问题是目前信息安全领域的研究热点之一。针对隐私保护下的分类问题,该文提出一种基于差分隐私保护的AdaBoost集成分类算法:CART-DPsAdaBoost(CART-Differential Privacy structure of AdaBoost)。算法在Boosting过程中结合Bagging的基本思想以增加采样本的多样性,在基于随机子空间算法的特征扰动中利用指数机制选择连续特征分裂点,利用Gini指数选择最佳离散特征,构造CART提升树作为集成学习的基分类器,并根据Laplace机制添加噪声。在整个算法过程中合理分配隐私预算以满足差分隐私保护需求。在实验中分析不同树深度下隐私水平对集成分类模型的影响并得出最优树深值和隐私预算域。相比同类算法,该方法无需对数据进行离散化预处理,用Adult、Census Income两个数据集实验结果表明,模型在兼顾隐私性和可用性的同时具有较好的分类准确率。此外,样本扰动和特征扰动两类随机性方案的引入能有效处理大规模、高维度数据分类问题。
Conference Papers
[NCMMSC] Xiaoyu Shen†, Wanyong Qiu†, Haojie Zhang, Yongzi Yu, Kun Qian*, Bin Hu*, "Self-Supervised Federated Learning for Heart Sound Recognition," in Proceedings of the 19th National Conference on Man-Machine Speech Communication (NCMMSC) , Urumq, China, August 2024 (EI).
PDF
Slides (Oral Report)
show/hide abstract
据2019全球疾病负担研究(Global Burden of Disease Study 2019, GBD)专题公布,2019年全球心血管疾病死亡人数约为1860万人,其中中国死亡人数约占比 1/4。因此,心血管疾病的早期诊断与日常监测至关重要。心音信号分析是对心血管疾病患者进行早期诊断与筛查的有效手段,但目前仍存在以下问题:数据安全与隐私限制了多源数据的中心化因而形成“数据孤岛”,同时精确标注的医疗健康数据极度稀缺。针对以上问题,本文提出应用自监督联邦学习模型实现心音异常检测,基于多中心化的心音数据集进行模型训练,在实现本地数据安全的同时,解决数据标签稀缺问题。实验验证了本文模型的有效性,并分析了联邦参与方数量对模型性能的影响。实验结果表明,在 PhysioNet心音数据集上,当参与方数量为2时模型的性能最优(准确率为65.04% ,非加权平均召回率为57.35%)。
[ICASSP] Yongzi Yu†, Wanyong Qiu†, Chen Quan, Kun Qian*, Zhihua Wang, Yu Ma, Bin Hu*, Björn W. Schuller, and Yoshiharu Yamamoto, "Federated Intelligent Terminals Facilitate Stuttering Monitoring," in Proceedings of IEEE International Conference on Acoustics, Speech and Signal Processing (ICASSP) , Rhodes Island, Greek, June 2023 (CCF B).
PDF
Slides (Oral Report)
show/hide abstract
Stuttering is a complicated language disorder. The most common form of stuttering is developmental stuttering, which begins in childhood. Early monitoring and intervention are essential for the treatment of children with stuttering. Automatic speech recognition technology has shown its great potential for non-fluent disorder identification, whereas the previous work has not considered the privacy of users data. To this end, we propose federated intelligent terminals for automatic monitoring of stuttering speech in different contexts. Experimental results demonstrate that the proposed federated intelligent terminals model can analyse symptoms of stammering speech by taking the personal privacy protection into account. Furthermore, the study has explored that the Shapley value approach in the federated learning setting has comparable performance to data-centralised learning.
[EMBC] Wanyong Qiu, Kun Qian*, Zhihua Wang, Yi Chang, Zhihao Bao, Bin Hu*, Björn W. Schuller, and Yoshiharu Yamamoto, "A Federated Learning Paradigm for Heart Sound Classification," in Proceedings of IEEE Engineering in Medicine & Biology Society (EMBC) , Glasgow, Scotland, UK, July 2022 (EI).
PDF
Slide (Poster)
show/hide abstract
Cardiovascular diseases (CVDs) have been ranked as the leading cause for deaths. The early diagnosis of CVDs is a crucial task in the medical practice. A plethora of efforts were given to the automated auscultation of heart sound, which leverages the power of computer audition to develop a cheap, non-invasive method that can be used at any time and anywhere for measuring the status of the heart. Nevertheless, previous works ignore an important factor, namely, the privacy of the user data. On the one hand, learnt models are always hungry for bigger data. On the other hand, it can be difficult to protect personal private information when collecting such large amount of data. In this dilemma, we propose a federated learning (FL) framework for the heart sound classification task. To the best of our knowledge, this is the first time to introduce FL to this field. We conducted multiple experiments, analysed the impact of data distribution across collaborative institutions on model quality and learning patterns, and verified the feasibility and effectiveness of FL based on real data. Non- independent identically distributed (Non-IID) data and model quality can be effectively improved by adding a strategy of globally sharing data.
Patents
[发明专利] 胡斌, 钱昆, 邱万勇 "一种基于联邦学习的心音-心电多模态融合检测方法[P]," 中国发明专利,申请号202411897434.6,2024年12月23日.
PDF
Slides
show/hide abstract
本发明公开了一种基于联邦学习的心音-心电多模态融合检测方法,旨在解决心血管疾病早期筛查和检测中的关键技术问题。首先提出多模态联邦医疗模型的分类、结构化定义和形式化表述。其次,利用跨模态协同机制,充分挖掘心音和心电数据之间的协同关系,以提升多医疗机构联邦检测模型的准确性和稳健性。
[发明专利] 胡斌, 邱万勇, 钱昆, "一种基于联邦学习的心音监测系统[P]," 中国发明专利,专利号ZL202211091843.8,授权公告日:2024年09月24日.
PDF
Slides
show/hide abstract
一种基于联邦学习的心音监测系统,其特征在于,所述系统包括联邦智能终端、联邦服务端、医疗云服务中心;所述联邦智能终端用于联邦学习系统的便携式智能心音感知,包括心音数据采集模块、心音数据预处理模块、心音特征提取模块、终端模型训练模块;所述联邦服务端用于协调各联邦参与方进行联合建模,包括模型参数安全聚合模块、全局模型更新模块;所述医疗云服务中心用于接收上传的稳定、可靠且具有可解释性的医疗模型并进行存储,以便各个基层医疗机构都可以下载使用。
[软件著作] 北京理工大学; 胡斌; 钱昆; 邱万勇, "面向智能体音感知的联邦学习智能客户端",计算机软件著作权,2023SR1107177,2023年09年19日.
PDF
Slides
show/hide abstract
面向智能体音感知的联邦学习智能终端是一款用于联邦医疗健康系统的便携式智能体音感知与分析终端。联邦智能终端包括体音数据采集功能模块、体音数据预处理功能模块、终端模型训练模块。在联邦服务器的协调下多中心的联邦智能终端可协作进行医疗建模,同时能有效解决数据安全与患者隐私保护的问题。
|